An online business strategy is a comprehensive plan that outlines how a business intends to operate, compete, and achieve its goals in the digital landscape. It is a roadmap that guides all aspects of the business, from marketing and sales to customer service and operations.
How does an online business strategy enable freedom to work from anywhere?
Definition of Online Business Strategy
An online business strategy is a plan that outlines how a business will leverage digital technologies to achieve its goals. It covers areas such as e-commerce, digital marketing, social media, content creation, and more. The strategy should be flexible and adaptable to changes in the digital landscape and customer behavior.
Benefits of Online Business Strategy
- Flexibility: An online business strategy allows businesses to operate from anywhere, providing employees with the freedom to work from home or a co-working space. This flexibility can improve employee satisfaction and productivity, leading to higher retention rates .
- Reach: An online presence can help businesses reach a wider audience, both locally and globally. This can lead to increased sales and customer engagement.
- Cost-effectiveness: Online business strategies can be more cost-effective than traditional business models. They eliminate the need for physical stores and reduce overhead costs associated with maintaining a physical presence.
Importance of Data Analysis in Online Business
Data analysis is a crucial component of an online business strategy. It involves collecting, analyzing, and interpreting data to understand customer behavior, market trends, and business performance. This information can help businesses make informed decisions, improve their online strategies, and achieve their goals f.
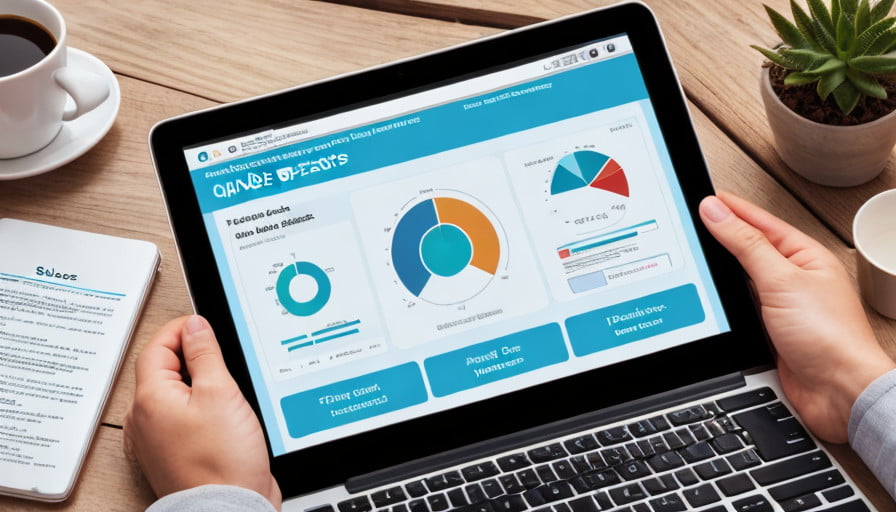
an online business strategy is a powerful tool that can enable freedom to work from anywhere, reach a wider audience, and make informed business decisions. By leveraging data analysis, businesses can gain valuable insights and drive their online success.
II. Overview of Data Analysis Techniques
Data analysis techniques are methods used to interpret and understand the data collected during research. They are crucial in drawing meaningful conclusions from the data.
Types of Data Analysis Techniques
Data analysis techniques can be broadly categorized into qualitative and quantitative. The choice between these two depends on the nature of the data and the research question at hand.
Qualitative Data Analysis Techniques
Qualitative data analysis techniques are used to interpret and understand non-numerical data. These techniques are useful when the research question requires understanding something (concepts, thoughts, experiences). Some common approaches to analyzing qualitative data include:
- Qualitative Content Analysis: This involves tracking the occurrence, position, and meaning of words or phrases in the data.
- Thematic Analysis: This method closely examines the data to identify the main themes and patterns.
- Discourse Analysis: This involves studying how communication works in social contexts.
Quantitative Data Analysis Techniques
Quantitative data analysis techniques are used to interpret and understand numerical data. These techniques are useful when the research question requires confirming or testing something (a theory or hypothesis). Quantitative data analysis techniques can include statistical analysis, regression analysis, and more.
Mixed Methods Research and Reviews
Mixed methods research is a research approach that combines both qualitative and quantitative methods. This approach is often used when the research question suggests that quantitative or qualitative data alone will not sufficiently answer the research question.
There are different types of mixed methods research designs, including exploratory sequential, parallel data analysis, and sequential synthesis design. The choice of design depends on the aim of the research, the timing of the data collection, and the importance given to each data type.
Data analysis techniques are essential tools in research, allowing researchers to draw meaningful conclusions from their data. The choice between qualitative, quantitative, or mixed methods research depends on the nature of the data and the research question.
III. Common Data Analysis Techniques
Data analysis techniques are methods used to interpret and understand the data collected during research. They are crucial in drawing meaningful conclusions from the data.
Thematic Analysis
Thematic analysis is a method of analyzing qualitative data. It is usually applied to a set of texts, such as an interview or transcripts. The researcher closely examines the data to identify common themes – topics, ideas, and patterns of meaning that come up repeatedly. This process involves familiarization, coding, generating themes, reviewing themes, defining and naming themes, and writing up.
Content Analysis
Content analysis is a research method for studying documents and including formats such as texts, interview transcripts, images, audio, or video. It involves systematically examining patterns in communication and discourse. This is done through a systematic reading or analysis of “texts” which are assigned codes to indicate the presence and proportion of meaningful content.
Statistical Analysis
Statistical analysis is a technique used to interpret and understand numerical data. It involves the use of statistical techniques to analyze and interpret data. This can include methods such as regression analysis, hypothesis analysis, and more.
Visual Analytics
Visual analytics is a data analysis technique that involves the use of visual methods to analyze data. This can include the use of charts, graphs, maps, and other visual representations to analyze and interpret data. Visual analytics can help to make complex data easier to understand and interpret.
Marker Selection Methods for High Throughput Data
Marker selection methods for high throughput data involve the use of specific markers or indicators to analyze and interpret high throughput data. This can include the use of specific algorithms or techniques to identify and analyze these markers.
Clustering and Labeling Procedures
Clustering and labeling procedures involve the use of specific techniques to group or categorize data. This can include the use of specific algorithms or techniques to identify and label these clusters. Clustering and labeling procedures can help to make complex data easier to understand and interpret.
In conclusion, data analysis techniques are essential tools in research, allowing researchers to draw meaningful conclusions from their data. The choice between these techniques depends on the nature of the data and the research question.
IV. Analyzing and Interpreting Data
Analyzing and interpreting data is a crucial part of any data-driven decision-making process. It involves understanding the data, identifying patterns, and drawing meaningful conclusions.
Planning for Data Analysis
Planning for data analysis involves several steps:
- Define the Problem: Clearly define the problem you are trying to solve or the question you are trying to answer. This will guide the rest of your data analysis process.
- Collect the Data: Identify the data sources that will be useful in answering your research question. This could involve collecting data from databases, surveys, interviews, or other sources.
- Prepare the Data: Clean and organize the data you’ve collected. This could involve removing duplicates, handling missing values, and converting data types.
- Analyze the Data: Use the appropriate data analysis techniques to interpret the data. This could involve statistical analysis, visual analytics, or other methods.
- Interpret the Results: Draw conclusions from your data analysis. This could involve identifying trends, making predictions, or answering your research question.
Demystifying Data Analysis
Data analysis can seem complex and overwhelming, but it’s a critical skill that can help you make better decisions. Here are some key points to understand about data analysis:
- Data Analysis is not just about finding patterns: While it’s important to identify patterns in the data, data analysis is also about understanding why these patterns exist. This involves interpreting the data and drawing meaningful conclusions.
- Data Analysis is iterative: Data analysis is not a one-time process. You will likely need to go back and forth between different steps of the data analysis process, refining your analysis as you go.
- Data Analysis requires critical thinking: Data analysis is not just about using tools and techniques. It requires critical thinking and the ability to interpret the data and draw meaningful conclusions.
Using Excel in Data Analysis
Microsoft Excel is a powerful tool for data analysis. It offers a wide range of features that can help you clean, organize, analyze, and visualize your data. Here are some ways you can use Excel in your data analysis:
- Data Cleaning: Excel provides various tools to clean and organize your data. This could involve using text functions, date and time functions, and conditional formatting.
- Data Analysis: Excel offers a variety of tools for analyzing your data. This could involve using pivot tables, subtotals with ranges, and other Excel functions.
- Data Visualization: Excel provides various tools for visualizing your data. This could involve creating charts, graphs, and other visual representations of your data.
In conclusion, planning for data analysis, understanding the basics of data analysis, and using tools like Excel can help you analyze and interpret your data more effectively. This can lead to better decision-making and improved outcomes.
V. Communicating and Reporting Data
Effective communication and reporting of data are critical in ensuring that the insights derived from data analysis are understood and acted upon by the relevant stakeholders.
Design Thinking for Data Science
Design thinking is a problem-solving approach that involves empathy, experimentation, and iteration. It can be effectively applied to data science projects to ensure that the solutions developed are not only technically sound but also meet the needs of the end users.
The design thinking process typically involves:
- Empathize: Understand the needs and perspectives of the end users.
- Define: Clearly define the problem and the user needs.
- Ideate: Generate a wide range of potential solutions.
- Prototype: Develop a simple version of the solution.
- Test: Get feedback on the prototype and iterate the design.
In the context of data science, design thinking can help ensure that the data science project is solving the right problem and that the solutions developed are practical and actionable.
Communicating and Reporting Data
Communicating and reporting data involves presenting the results of data analysis in a clear, concise, and understandable manner. This can involve using various techniques and tools, such as:
- Data Visualization: Data visualization involves using graphs, charts, and other visual representations to present data. It can help stakeholders understand complex data and identify trends and patterns.
- Storytelling: Storytelling involves presenting data as a narrative. It can help stakeholders understand the context of the data and the implications of the findings.
- Data Table: Data tables are a simple and effective way to present data. They can be used to present raw data or to summarize data.
- Presenting Data for Impact: When presenting data, it’s important to keep the audience in mind. The data should be presented in a way that is easy to understand and that clearly explains the findings.
In conclusion, design thinking can help ensure that data science projects are user-focused, while effective communication and reporting of data can help ensure that the insights derived from data analysis are understood and acted upon by the relevant stakeholders.
VI. Conclusion
Summary of Key Points
Throughout this discussion, we’ve covered a wide range of topics related to online business strategies and data analysis techniques. We’ve explored the definition and importance of online business strategies, the benefits of these strategies, and the role of data analysis in online businesses. We’ve also delved into various data analysis techniques, including thematic analysis, content analysis, statistical analysis, visual analytics, marker selection methods for high throughput data, and clustering and labeling procedures.
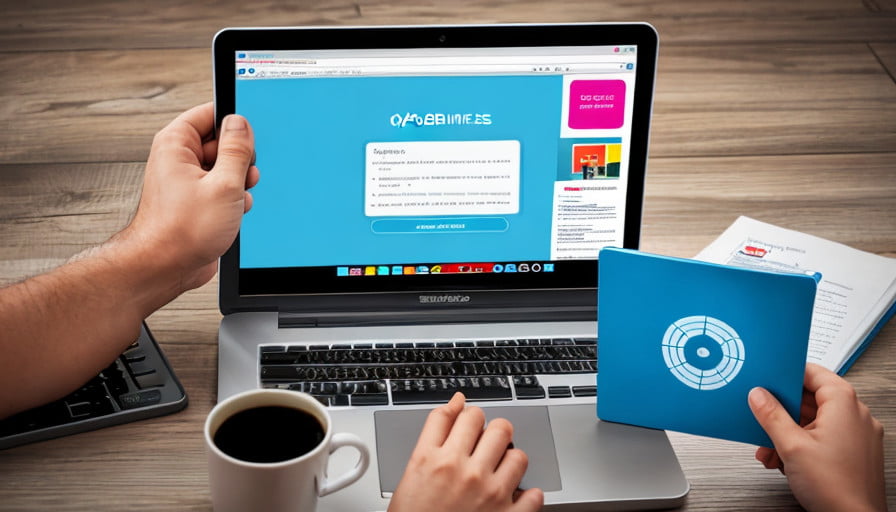
We’ve also discussed the planning and interpretation of data, the use of Excel in data analysis, and the importance of communicating and reporting data effectively. Finally, we’ve touched upon the concept of design thinking for data science and its application in data communication and reporting.
Future Directions for Online Business Strategy and Data Analysis Techniques
Looking ahead, the future of online business strategies and data analysis techniques is promising. With the continuous advancement in technology and the increasing digitization of businesses, online business strategies will become even more critical. They will enable businesses to reach a wider audience, improve customer engagement, and achieve their goals more effectively.
In terms of data analysis techniques, we can expect to see the continued development and application of advanced techniques. This includes the use of artificial intelligence and machine learning in data analysis, which can help to automate and improve the accuracy of data analysis.
Moreover, the importance of data communication and reporting will continue to grow. As businesses become more data-driven, the ability to effectively communicate and report data will become even more critical. This will involve not only presenting the data in a clear and understandable manner, but also using data visualization and storytelling techniques to engage and influence the audience.
In conclusion, the future of online business strategies and data analysis techniques holds great potential for businesses. By leveraging these strategies and techniques, businesses can gain a competitive edge, improve their decision-making, and achieve their goals more effectively. In order to learn more, read another article i wrote about >>>> Strategic Triumph: Uncovering the Untold Benefits of Crafting an Online Business Strategy to learn more.

As an author writing and creating business courses and articles, I am responsible for developing and delivering high-quality content that is informative, engaging, and relevant to the target audience.
I monitor and analyzes business trends and topics to create courses and articles that provide value to readers and students here. I am responsible for ensuring that all content is accurate, well-written, and met the needs of the target audience.