In the digital age, businesses are increasingly leveraging online platforms to reach their customers. With the advent of e-commerce, the importance of online business strategies in improving customer support has never been more critical. Online business strategies can significantly enhance the customer experience, leading to increased customer satisfaction and loyalty. This article will delve into how effective online business strategies can improve quality customer support.
Firstly, online business strategies can help improve customer service by enhancing the customer journey. This can be achieved by providing multiple channels for customer interaction, such as email, live chat, and social media. These channels allow businesses to engage with customers in real-time, providing instant support and resolving issues quickly.
Secondly, online business strategies can help improve customer support by empowering customers to serve themselves. By providing resources such as FAQs, guides, and tutorials, businesses can enable customers to find answers to their questions and resolve issues without needing to contact customer support.
Thirdly, online business strategies can help improve customer support by personalizing the customer experience. By collecting and analyzing customer data, businesses can provide personalized recommendations, offers, and support, enhancing the customer experience and increasing customer satisfaction.
Lastly, online business strategies can help improve customer support by fostering a culture of customer-centricity. This involves prioritizing customer needs and feedback, and continually seeking ways to improve the customer experience. By doing so, businesses can build strong customer relationships, leading to increased customer loyalty and repeat business h.
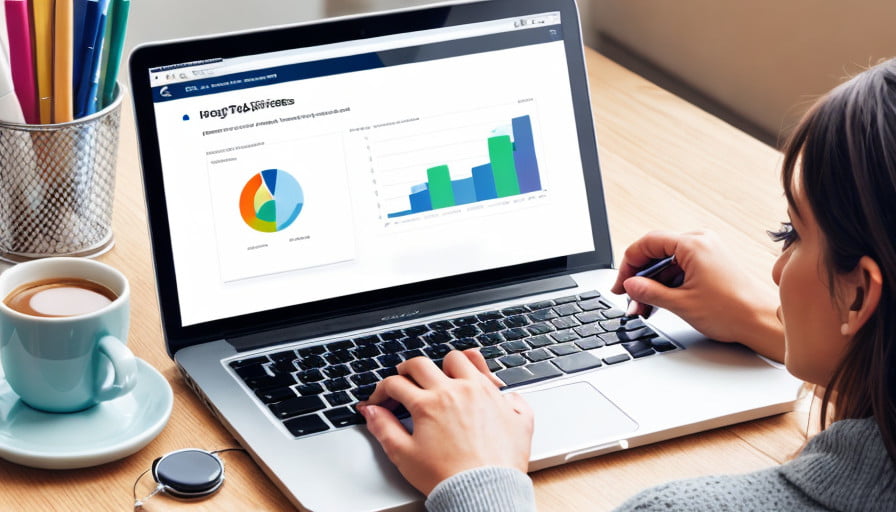
online business strategies play a crucial role in improving customer support. By enhancing the customer journey, empowering customers, personalizing the customer experience, and fostering a customer-centric culture, businesses can provide superior customer support and create a positive customer experience.
How can online business strategy improve quality customer support?
Online business strategies play a pivotal role in enhancing the quality of customer support. By leveraging digital platforms, businesses can effectively reach out to customers, address their concerns, and provide timely resolutions. This can be achieved through various strategies such as:
- Customer Service Channels: Businesses can utilize multiple channels like email, social media, live chat, and phone calls to interact with customers. This multi-channel approach ensures that customers are always within reach and their queries are addressed promptly.
- AI and Automation: AI-powered chatbots can handle routine queries, freeing up human agents to focus on complex issues. Automation can also streamline processes, reducing response times and improving customer satisfaction.
- Customer Feedback: Regularly soliciting and acting upon customer feedback can significantly improve customer support. Businesses can use online surveys and feedback forms to gather insights and make necessary improvements.
Introduction to Data Analysis Techniques
Data analysis techniques are crucial tools in today’s data-driven world. They allow businesses to extract meaningful insights from their data, enabling informed decision-making and strategic planning. These techniques can be broadly categorized into:
- Descriptive Statistics: This technique involves summarizing and organizing data to provide a simple picture of the sample or population. It includes measures like mean, median, mode, range, etc.
- Inferential Statistics: This technique involves making predictions or inferences about a population based on a sample. It includes methods like hypothesis testing, regression analysis, etc.
- Predictive Modeling: This technique involves using historical data to forecast future trends. It includes methods like regression, decision trees, etc.
- Machine Learning: This is a subset of AI that provides systems the ability to learn and improve from experience without being explicitly programmed. It includes techniques like clustering, neural networks, etc.
Types of Data Analysis Techniques
Data analysis techniques come in various forms, each suited to different types of data and analysis needs. Here are some common types:
- Qualitative Data Analysis: This involves analyzing non-numerical data like text, audio, images, etc. Techniques include content analysis, thematic analysis, etc.
- Quantitative Data Analysis: This involves analyzing numerical data. Techniques include descriptive statistics, inferential statistics, predictive modeling, etc.
- Mixed Methods Analysis: This involves combining qualitative and quantitative data analysis. It provides a more comprehensive understanding of the research problem.
- Exploratory Data Analysis (EDA): This involves investigating the data to discover patterns, spot anomalies, test hypotheses, and check assumptions. It’s a crucial step before any data analysis.
Inferential Statistics
Inferential statistics is a branch of statistics that deals with the application of probability theory to statistics. It involves making inferences about a population based on a sample from that population. This is achieved through various methods such as hypothesis testing, confidence intervals, and prediction intervals.
- Hypothesis Testing: This involves making a decision about a population parameter based on the data sampled from that population. The decision is usually framed in terms of rejecting or failing to reject a null hypothesis.
- Confidence Intervals: This is a range of values, derived from a statistical procedure, that is likely to contain the value of an unknown population parameter.
- Prediction Intervals: These are used to predict the value of an unknown population parameter in the future.
Regression Analysis
Regression analysis is a statistical technique used to understand the relationship between dependent and independent variables. It involves estimating the coefficients of the regression equation, which describe the relationship between the independent and dependent variables.
- Linear Regression: This is the most common type of regression analysis. It models the relationship between two variables by fitting a linear equation to observed data.
- Logistic Regression: This is used when the dependent variable is binary. It models the probability that each input point belongs to a certain category.
- Polynomial Regression: This is a type of regression analysis in which the relationship between the independent variable x and the dependent variable y is modeled as an nth degree polynomial.
Correlation Analysis
Correlation analysis is a statistical method used to evaluate the strength and direction of the relationship between two or more variables. It provides a statistical measure of the relationship between two or more variables, helping to understand how one variable may change in relation to another.
- Pearson Correlation: This is the most common type of correlation analysis. It measures the linear relationship between two continuous variables.
- Spearman’s Rank Correlation: This is a non-parametric measure that assesses how well the relationship between two variables can be described using a monotonic function.
- Kendall’s Tau: This is another non-parametric correlation measure used to detect the strength of dependence between two variables.
Time Series Analysis
Time series analysis is a statistical technique used to analyze time series data, or trend analysis. It involves the use of various methods to extract meaningful statistics and other characteristics of the data.
- Autocorrelation: This is the correlation between two observations at different points in a time series. For example, values that are separated by an interval might have a strong positive or negative correlation.
- Moving Averages: These can smooth time series data, reveal underlying trends, and identify components for use in statistical modeling.
- Stationarity: A time series is said to be stationary if its properties do not depend on the time at which the series is observed. This is a crucial assumption in many time series models and methods.
Cluster Analysis
Cluster analysis is a method of statistical analysis used to classify a set of n objects into k groups or clusters. The objects are usually similar in some way, and the grouping is based on the similarity of the objects.
- K-means Clustering: This is one of the most common methods for clustering. It works by initializing k centroids randomly, assigning each data point to the nearest centroid, and then updating the centroids by calculating the mean of all data points assigned to each centroid.
- Hierarchical Clustering: This method builds a hierarchy of clusters by either a bottom-up or top-down approach. In the bottom-up approach, each data point starts in its own cluster, and pairs of clusters are merged as one moves up the hierarchy.
- DBSCAN (Density-Based Spatial Clustering of Applications with Noise): This method groups together points that are packed closely together (points with many nearby neighbors), marking as outliers points that lie alone in low-density regions.
Factor Analysis
Factor analysis is a statistical method that aims to describe a set of correlated variables in terms of a smaller number of uncorrelated variables called factors. It is used when the variables are interrelated and the aim is to understand the relationships among these variables.
- Common Factor Analysis (CFA): This is used when the underlying process or construct is involved. It analyzes only the reliable common variance of data. CFA is often used when the purpose is to explain correlations among variables and to examine the structure of the data.
- Principal Component Analysis (PCA): This is used when the underlying process or construct is not involved. It analyzes all the variance of data. PCA is often used when the purpose of a study is to summarize data with a smaller number of variables.
Principal Component Analysis
Principal Component Analysis (PCA) is a technique used to emphasize variation and bring out strong patterns in a dataset. It’s often used to make data easy to explore and visualize.
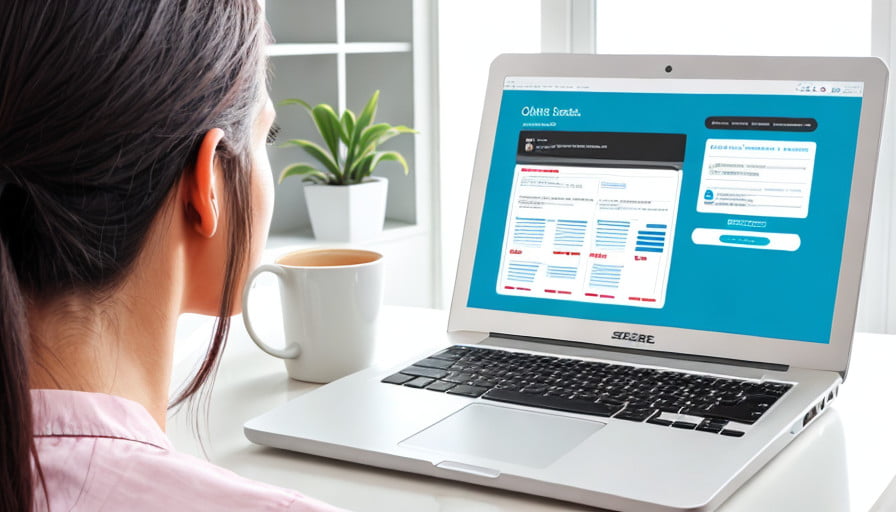
- Standardization: PCA is affected by the scales of the variables. Hence, it is a common practice to bring all variables to a similar scale.
- Computing Covariance Matrix: The covariance matrix is used to understand the variance and covariance between the different variables.
- Eigenvalues and Eigenvectors: The eigenvectors and eigenvalues of the covariance matrix are used to transform the original variables to a new set of variables.
- Sorting and Selecting Principal Components: The principal components are sorted by their corresponding eigenvalues. The first few principal components that retain a significant amount of variance in the data are selected.
- Transforming the Original Data: The original data is then transformed using the selected principal components.
Data Mining Techniques
Data mining is the process of discovering patterns in large data sets involving methods at the intersection of machine learning, statistics, and database systems. It’s an essential tool in data analysis that can help businesses make sense of their data.
- Frequent Itemset Analysis: This technique is used to discover associations among a set of transactions. It’s commonly used in market basket analysis, where the goal is to find associations between different products that customers buy together.
- Communities: This technique is used to identify groups or communities within a network. It’s often used in social network analysis to identify groups of individuals who are connected to each other.
- Sampling Data in a Stream: This technique is used to select a subset of a data stream so that we can ask queries about the deleted subset and have the answers to be statistically representative of the stream as a whole.
- Social Network Graphs: This technique is used to model social networks as graphs. The entities are the nodes, and an edge connects two nodes if the nodes are related by the relationship that characterizes the network.
Machine Learning Techniques
Machine learning is a type of artificial intelligence that provides systems the ability to learn and improve from experience without being explicitly programmed. It focuses on the development of computer programs that can access data and use it to learn for themselves.
- Neural Networks: These are algorithms that mimic the human brain—albeit far from matching its ability—in order to recognize relationships in vast amounts of data. They interpret sensory data through a kind of machine perception, labeling or clustering raw input.
- Decision Trees: This technique uses classification or regression methods to classify or predict potential outcomes based on a set of decisions. It uses a tree-like visualization to represent the potential outcomes of these decisions.
- K-Nearest Neighbor (KNN): This is a non-parametric algorithm that classifies data points based on their proximity and association to other available data. It calculates the distance between data points, usually through Euclidean distance, and then it assigns a category based on the most frequent category or average.
Artificial Intelligence Techniques
Artificial Intelligence (AI) refers to the simulation of human intelligence processes by machines, especially computer systems. These processes include learning, reasoning, problem-solving, perception, and language understanding.
- Deep Learning: This is a subset of machine learning that is inspired by the structure and function of the brain. It uses neural networks with many layers (hence the term “deep”) to model and understand complex patterns.
- Supervised Learning: This is a type of machine learning where the model is trained on a labeled dataset. The model learns to predict the output from the input data.
- Unsupervised Learning: This is a type of machine learning where the model is trained on an unlabeled dataset. The model learns to identify patterns and relationships in the data without any prior training.
Text Analytics Techniques
Text analytics is a method of analyzing a large amount of text data to discover patterns, themes, and trends. It’s used in various fields like marketing, healthcare, and social media to extract insights from unstructured text data.
- Sentiment Analysis: This technique involves determining the sentiment or emotion expressed in a piece of text. It’s often used in social media monitoring to understand public opinion about a brand, product, or event.
- Topic Modeling: This involves discovering the main topics in a collection of documents. Latent Dirichlet Allocation (LDA) is a popular technique used for topic modeling.
- Natural Language Processing (NLP): This is a subfield of AI that focuses on the interaction between computers and humans through natural language. It includes techniques like tokenization, stemming, and lemmatization.
- Text Classification: This involves categorizing text into predefined categories. It’s often used in spam detection, customer service, and content moderation.
Social Network Analysis
Social network analysis (SNA) is a method used to study relationships within and between social networks. It involves the examination of structures, dynamics, and functions of social networks.
- Node Degree: This is the number of edges connected to a node in a network. In an undirected network, there’s only one measure for degree. However, in a directed network, there are three different degree measures: in-degree, out-degree, and degree.
- Edge Weight: This is the number of times an edge appears between two specific nodes. For example, if person A buys a coffee from a coffee shop three times, the edge connecting person A and the coffee shop will have a weight of three.
- Network Size: This is the number of nodes in the network. The size of a network does not take into consideration the number of edges.
- Hubs and Authorities: Hubs are nodes that have many edges pointing out of them, while authorities are nodes that have many edges pointing to them.
Big Data Analytics Techniques
Big data analytics is the process of examining and interpreting complex data sets to discover useful information, draw conclusions, and support decision-making. It involves various techniques to handle and analyze big data.
- Data Cleaning: This involves removing or modifying corrupted data to ensure the accuracy and reliability of the dataset.
- Data Integration: This involves combining data from different sources to provide a unified view of the data.
- Data Mining: This involves discovering patterns and insights in large datasets using various machine learning techniques.
- Predictive Analytics: This involves using statistical algorithms and machine learning techniques to identify future trends based on historical data.
- Real-Time Analytics: This involves analyzing data in real-time to provide immediate insights and support decision-making.
Data Visualization Techniques
Data visualization is the graphical representation of data and information. It involves the use of graphical elements like charts, graphs, and maps to represent data in a way that is easy to understand.
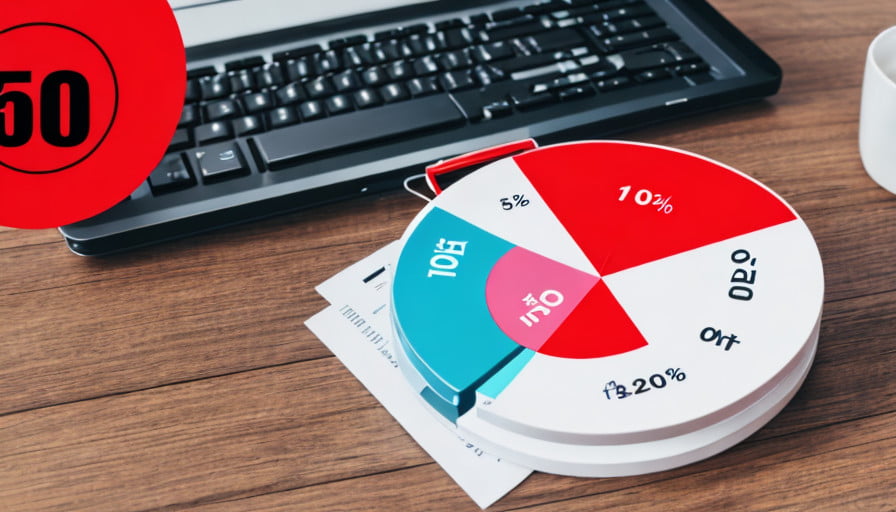
- Bar Charts: These are used to compare the magnitude of different categories of data. They can be horizontal or vertical, depending on the nature of the data.
- Line Graphs: These are used to show trends over time. They are useful for comparing the same category of data over different periods.
- Pie Charts: These are used to show the proportion of different categories of data. They are useful when the total amount is important.
- Scatter Plots: These are used to show the relationship between two numerical variables. They are useful for identifying trends, correlations, and outliers.
- Heat Maps: These are used to represent data in a two-dimensional matrix format, where individual values are represented by colors.
Data Reporting Techniques
Data reporting is the process of presenting data in a structured and understandable format. It involves summarizing data, presenting it in a readable format, and interpreting the results.
- Summary Reports: These are used to provide a quick overview of the data. They summarize the key metrics and trends in the data.
- Detail Reports: These are used to provide a more detailed view of the data. They include more granular data and can be used to analyze specific segments or periods.
- Trend Reports: These are used to analyze and report on trends in the data. They identify patterns and changes over time.
- Comparison Reports: These are used to compare different sets of data. They can be used to analyze performance, compare different groups, or compare different periods.
- Predictive Reports: These are used to forecast future trends based on historical data. They use statistical and machine learning techniques to make predictions.
Data Quality Assessment Techniques
Data quality assessment is the process of evaluating the quality of data. It involves checking the accuracy, consistency, reliability, and completeness of the data.
- Data Profiling: This involves analyzing the data to understand its characteristics, such as the distribution of values, the presence of outliers, and the relationships between variables.
- Data Validation: This involves checking the data against predefined rules or criteria to ensure its accuracy and consistency.
- Data Cleansing: This involves identifying and correcting errors in the data, such as missing values, inconsistent formats, and incorrect values.
- Data Redundancy Check: This involves checking the data for redundancy, such as duplicate records and unnecessary duplication of data.
Data Governance Techniques
Data governance is the practice of managing the availability, usability, integrity, and security of data. It involves defining policies, establishing processes, and assigning roles and responsibilities for managing data.
- Data Governance Policy: This is a document that outlines how data will be managed and controlled. It covers areas like data quality, data availability, data usability, data integrity, and data security.
- Data Governance Roles: These are the roles and responsibilities assigned for managing data. They include roles like Chief Data Officer, Data Governance Manager, and Data Governance Committee.
- Data Governance Maturity Model: This is a model used to evaluate the maturity of data governance practices. It includes levels like Unaware, Proactive, and Effective.
- Data Governance Strategy: This involves defining the content of the data governance framework. It includes defining important entities, the current and desired quality of the data, and the requirements for the data to meet the goals.You should read another article i wrote about >>>>
- Strategic Triumph: Uncovering the Untold Benefits of Crafting an Online Business Strategy to learn more.
- Sarah Shane

As an author writing and creating business courses and articles, I am responsible for developing and delivering high-quality content that is informative, engaging, and relevant to the target audience.
I monitor and analyzes business trends and topics to create courses and articles that provide value to readers and students here. I am responsible for ensuring that all content is accurate, well-written, and met the needs of the target audience.